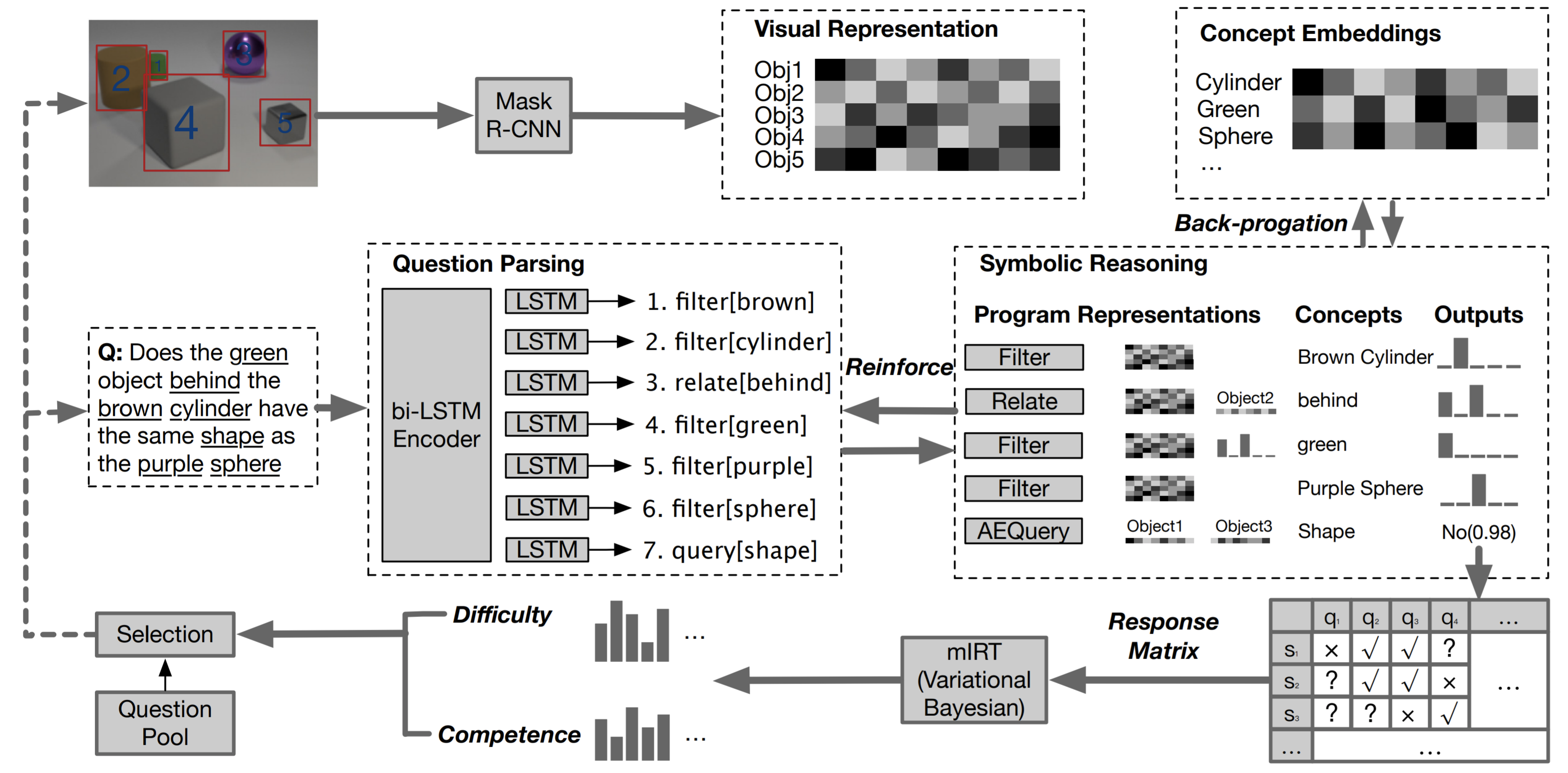
Humans can progressively learn visual concepts from easy to hard questions. To mimic this efficient learning ability, we propose a competence-aware curriculum for visual concept learning in a question-answering manner. Specifically, we design a neural-symbolic concept learner for learning the visual concepts and a multi-dimensional Item Response Theory (mIRT) model for guiding the learning process with an adaptive curriculum. The mIRT effectively estimates the concept difficulty and the model competence at each learning step from accumulated model responses. The estimated concept difficulty and model competence are further utilized to select the most profitable training samples. Experimental results on CLEVR show that with a competence-aware curriculum, the proposed method achieves state-of-the-art performances with superior data efficiency and convergence speed. Specifically, the proposed model only uses 40% of training data and converges three times faster compared with other state-of-the-art methods.